By Stelia’s Chief Marketing Officer, Paul Morrison
The competition for GPU chips and then for data centre capacity is well documented and ongoing. However, the elephant in the room is the future volume of data generated by GPU clusters. The ability to move that data is frequently downgraded to afterthought status in the current scramble for available power resources.
Whilst it is tempting to “fix the network later”, later may never come if your business model is shackled by sub-optimal connectivity while your competition has taken a more nuanced view.
Optimised Network Accelerates ROI
According to Ronen Dar, CTO and co-founder of Run:AI, latency can have significant cost impacts on AI workloads, particularly in inference scenarios. “Latency and throughput are big challenges when it comes to inference, and it impacts the cost of AI, and the cost of AI right now is very high.”
Ronen is politely inferring than an optimised network is a prerequisite for an optimised return on investment. Or a better network is directly correlated to financial performance.
Eliminating latency – as far as the laws of physics allows – between colocation, GPU clouds, storage platforms, on-premises and public clouds is one of several data mobility success factors. Eliminating latency will maximise all subsequent efforts to optimise performance.
The Full Impact of Latency
High latency is more than just inconvenient – it’s a significant bottleneck with wide-ranging impacts. Here, we explore how high latency can affect GPU utilisation, infrastructure provisioning, business opportunities, operational complexity, scalability, and user experience.
Stelia identifies at least six significant business issues arising from latency which will significantly undermine the return from AI investments as:
– Underutilised GPU Resources – the GPU CSP Dilemma
When inference requests experience high latency, GPUs often sit idle, waiting for data or results to be transferred. This downtime reduces GPU utilisation, leading to wasted resources. Idle GPUs contribute to higher operational costs without delivering proportional value, making efficient resource management crucial for cost-effective AI operations.
– Overprovisioning of Infrastructure
To mitigate the effects of high latency and maintain acceptable response times, organisations frequently overprovision their AI infrastructure. This involves allocating more GPU resources than the workload necessitates. While this approach may help performance, it also results in substantial unnecessary expenses. Efficiently balancing resource allocation without overprovisioning remains a key challenge for organisations aiming to optimise costs.
– Missed Business Opportunities
High latency can slow down AI-driven decision-making processes, adversely impacting business operations. In sectors such as finance, healthcare, and e-commerce, real-time AI insights are critical. Delays can lead to missed opportunities, lost revenue, and competitive disadvantages. Rapid and reliable AI response times are essential for capitalising on these insights and maintaining a competitive edge.
– Increased Operational Complexity
Minimising latency in AI systems is a complex and time-consuming task. Organisations need to manage and optimise their infrastructure continually, often requiring specialised expertise, tools, and processes. This added complexity increases operational costs and can divert resources from other critical business functions. Streamlining operations to handle latency effectively is a strategic necessity.
– Scalability Challenges
As AI workloads expand and the demand for real-time inference grows, high latency can hinder the scalability of AI systems. Organisations may find it challenging to scale their infrastructure efficiently to meet increasing demands, leading to performance degradation and higher costs. Scalability solutions must address latency to ensure seamless growth and sustained performance.
– Negative User Experience
For consumer-facing AI applications, high latency translates directly into poor user experiences. Slow response times and delayed outputs can frustrate users, leading to decreased engagement, customer churn and reputational damage. In competitive markets, a negative user experience can quickly result in lost revenue and diminished brand loyalty. Ensuring fast and reliable AI interactions is vital for maintaining user satisfaction and business success.
To conclude, addressing latency is critical for optimising GPU utilisation, managing infrastructure costs, seizing business opportunities, reducing operational complexity, scaling effectively, and enhancing user experience. Organisations that prioritise low-latency AI systems will position themselves better to harness the full potential of their AI investments.
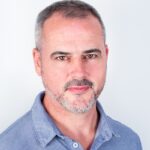