By Stelia’s Chief Marketing Officer, Paul Morrison
Part of the $100 Trillion industrial revolution forecast by NVIDIA CEO Jensen Huang is driven by machine-to-machine (M2M) communications. The synergy between M2M systems and AI workloads has become a necessity for innovation across industries.
However, legacy networking protocols and a lack of specialized Network Operations (NetOps) focus have given rise to significant data distribution inefficiencies. These inefficiencies hamper AI workload performance, escalate operational complexity, and ultimately impact business outcomes.
This is an aspect of the larger data mobility challenge.
The Symbiotic Relationship Between M2M and AI
M2M communication and AI workloads are intrinsically linked through various mechanisms:
1. Data Generation: M2M systems generate vast volumes of data from diverse sources, such as manufacturing equipment, smart devices, and autonomous vehicles. This data is essential for training AI models and enabling AI-driven analytics and decision-making.
2. Predictive Maintenance: AI algorithms leverage M2M data streams to predict maintenance needs, reducing downtime and enhancing efficiency in sectors reliant on M2M systems.
3. Autonomous Systems: AI facilitates the functioning of autonomous systems, including self-driving vehicles, by processing real-time data from interconnected sensors and cameras.
4. Edge Computing: The proliferation of M2M devices necessitates edge computing to process data closer to its source, reducing latency and bandwidth use. AI workloads at the edge support real-time decision-making.
5. Data Analytics: M2M systems’ data requires advanced analytics, provided by AI, to extract insights, identify patterns, and optimize processes.
This interdependence between M2M and AI is driving digital transformation, but it also highlights an urgent need for efficient data mobility.
The Challenge of Legacy Protocols
In the age of AI, data mobility—defined as the efficient movement and accessibility of data across distributed networks and systems—is paramount. Legacy protocols like MPLS, CoS, QoS, and MEF, designed for human-centric traffic, fall short in optimizing M2M communication, leading to several business challenges:
1. Suboptimal Performance: Legacy protocols often fail to prioritize M2M communication, resulting in latency, jitter, and packet loss, degrading AI workload performance and accuracy. The inability to seamlessly move large datasets quickly between on-premises, colocation, GPU clouds, public clouds and storage platforms impedes AI model training and real-time analytics.
2. Inefficient Bandwidth Utilization: Traditional traffic engineering and QoS mechanisms do not effectively allocate bandwidth for M2M traffic, causing underutilization or congestion. This inefficient bandwidth use limits the scalability of AI applications and drives up costs, as more resources are required to achieve desired performance levels.
3. Real-Time Requirements: AI workloads rely on real-time M2M communication. Legacy protocols struggle to provide the low-latency, deterministic performance required, leading to suboptimal outcomes and potential safety risks in critical applications, such as autonomous vehicles or industrial automation.
4. Limited Visibility and Control: Traditional network monitoring tools lack the granularity needed for M2M traffic, complicating performance issue identification, routing optimization, and reliable AI workload delivery. Without clear insights into data flow, optimizing AI-driven processes becomes a significant challenge.
5. Increased Complexity and Costs: Adapting legacy protocols to M2M needs results in complex configurations, manual interventions, and higher operational overhead. The increased complexity not only drives up costs but also reduces organizational agility, making it harder to respond swiftly to evolving business needs.
Limitations of MPLS and IP Protocols
Massive AI workloads, driven by M2M communication, challenge traditional networking protocols like MPLS and IP due to their unique needs for ultra-low latency, high bandwidth, and dense port connectivity.
Impact on MPLS:
– MPLS, designed for efficient routing in enterprise WANs, struggles with AI workloads requiring ultra-low latency and high bandwidth.
– The overhead and routing complexities of MPLS make it difficult to achieve the microsecond-range latencies needed by AI.
– AI infrastructure’s reliance on parallel processing with numerous GPUs necessitates dense port connectivity, which MPLS networks cannot provide efficiently.
Impact on IP Protocols:
– Traditional IP routing is overwhelmed by the volume and size of AI data flows.
– IP routing tables and path selection algorithms are not optimized for AI traffic’s low-latency, high-throughput demands.
– Configuration and management errors in traditional IP networks can severely impact AI workload performance.
Conclusion
While MPLS and IP protocols have served enterprise networking needs well, the unique demands of massive AI workloads are driving the adoption of more optimized networking solutions tailored specifically for AI infrastructure.
The focus on improving data mobility is essential to unlock the full potential of AI applications, ensuring efficient data distribution and robust performance of M2M-driven processes.
By working with forward-looking data mobility providers to address these challenges, organizations can significantly enhance operational efficiency and achieve better business outcomes.
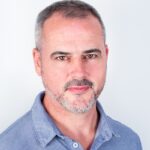